Why AIClub Research Program?
The only program that enables your student to build unique research projects on a topic they care about.
Topics from science, healthcare, climate, sports, music, and more.
Expert mentors with Ph.Ds in computer science and artificial intelligence.
Proven wins with 200+ national and international awards and 25+ research publications in the past 2 years alone. Six patents were submitted by students using their AIClub projects!
Personalized Program
-
One-on-one mentorship
-
Flexible scheduling
-
Leverage your academic interests
-
Online from anywhere
Expert Mentors
-
Work one-on-one with a researcher in the field
-
Receive personal feedback and advice from an academic expert
Unique Projects
-
Submit to competitions
-
Present at a conference
-
Publish a paper
-
Create an app
-
Build hardware powered by AI

Welcome to our research institute, where we offer a unique approach to learning and executing unique research projects.
Unlike typical matching services that merely pair you with a researcher and step back, we stand out as a comprehensive platform dedicated to your growth. With a team of accomplished PhD mentors and skilled instructors, we're committed to guiding you through a journey of discovery.
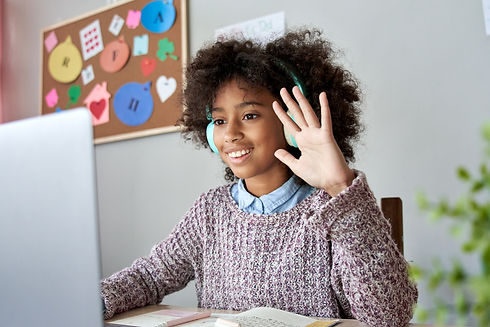
Project Spotlight
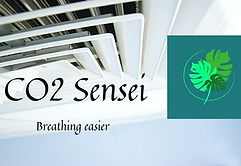
CO2 Sensei
The pandemic directly affected the way businesses operate, threatening many people's livelihoods. Team MDS created an iPhone app that businesses and individuals can use to measure the CO2 levels around them, to help them in reopening their business.
Awards
1. Technovation Girls Grand Prize 2021
2. Project CS Girls National Winner 2021
3. Congressional App Challenge - Gift Certificate and Presentation Opportunity - Jackie Speier District 2021
4. Poster Presentation accepted to the ICML COVID/AI Workshop 2022

Climate Change
Projects to tackle the issue of the prominent threat of climate change are rising. This project uses AI to detect and measure greenhouse gases more effectively.
Awards
1. Alameda County Science Fair - 1st Place 2022
2. Special award from USAID
2022
3. CSEF - 4th Place Winner in Senior Division 2022
4. JSHS National Symposium -1st Place Poster Category in Environmental Sciences 2022
5. Paper accepted into AAAI 2022
6. Two papers accepted to the MIT Student Research Conference 2022
7. BioGENEius - Finalist 2022
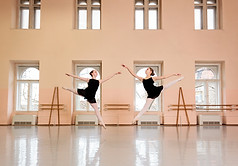
AI Ballet Instruction
AI in combination with the fine arts can produce unique and interesting innovations. This student trained her AI to understand and teach ballet to students. This is a great option for many aspiring students who may not find ballet lessons accessible or affordable. This research project included innovative uses of AI to analyze video, followed by classification techniques to assess the quality of dance moves. The solution is capable of analyzing dance moves and recommending corrections for correct execution and physical safety.
Awards
1. Research paper accepted to the 21st IEEE International Conference on Machine Learning Applications - Special Session on Education (2022). The research will be published in the conference proceedings and presented live to workshop attendees.
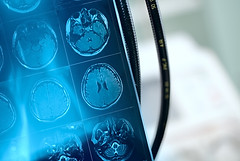
Alzheimer's is one of the top 10 causes of death in the United States and is currently incurable. This student created an app that allows for a user to insert an MRI image, and the AI will predict the risk of Alzheimer's from Low to High risk. This AI helps with the diagnosis of Alzheimer's at an earlier stage, thereby allowing patients to try different treatment options and encouraging a more positive patient outcome.
Awards
1. Synopsys Science and Technology Championship (Santa Clara Valley Engineering Fair Association) - Special Award from the Army and Navy Corps - 2021
2. Research paper published at SPIE Workshop on Machine Learning 2022. Paper was presented live to conference attendees and appears in the SPIE 2022 Conference Proceedings.
3. Pending Patent 2022
Forecasting Alzheimers
Select Publications from Past Students
View more
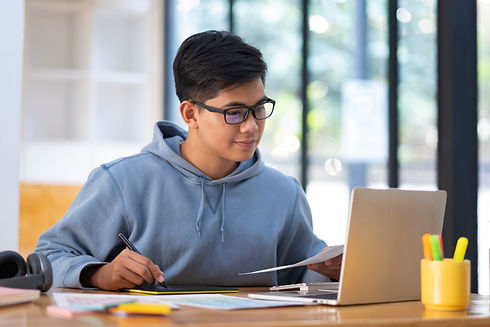
Our high-quality curriculum is designed to empower you to craft your research projects from scratch. We believe in the value of learning by doing, and our approach ensures that you not only understand theories but also put them into action in a research project.
But we don't stop at learning. We're passionate about showcasing your research to the world. We support you in publishing your work at various competitions and esteemed venues. Your research deserves to be recognized, and we're here to help you shine.
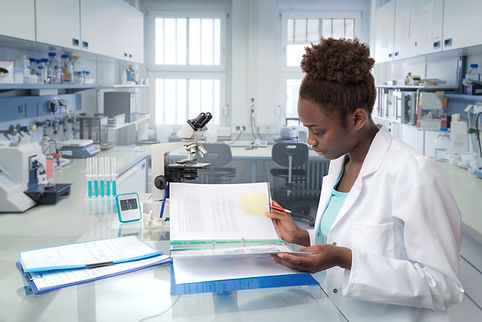
Join us on a transformative research journey where mentors, instructors, and a vibrant community come together to elevate your skills, empower your creativity, and celebrate your accomplishments.
Welcome to a place where learning meets execution and knowledge transforms into meaningful contributions.
How does the Research Program work?

Begin at any time of the year, with the flexibility to choose a schedule and frequency that suits your needs. Most students opt for 1-2 hours of sessions per week. Enjoy free cancellation and rescheduling with just a 3-day notice. No upfront fees—simply pay as you go, making it both easy and convenient to participate.
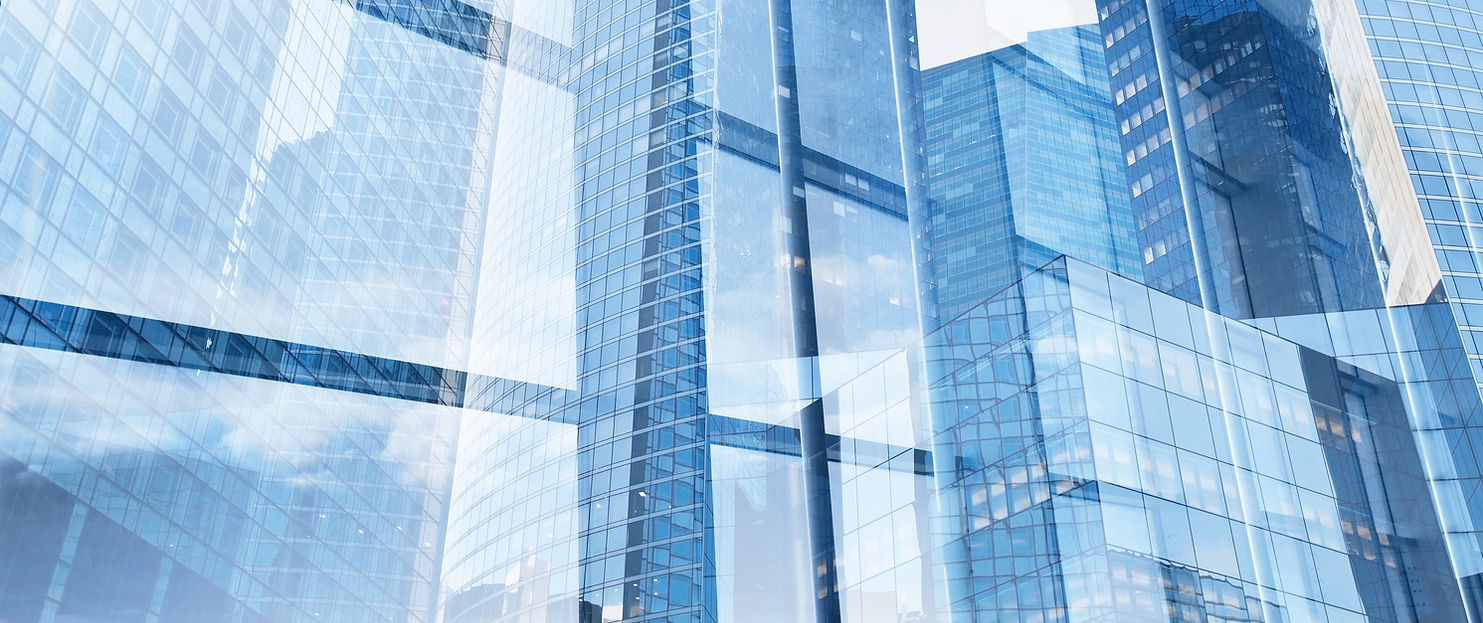
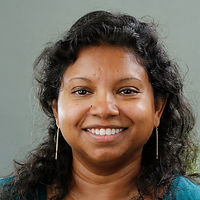
Dr.Nisha Talagala
Nisha Talagala is the CEO and founder of AIClub. Nisha co-founded ParallelM which pioneered the MLOps practice of managing machine learning in production. Nisha is a recognized leader in the operational ML space with more than 20 years of expertise in software development, distributed systems, technical strategy and product leadership. Nisha earned her PhD at UC Berkeley where she did research on clusters and distributed systems. Nisha co-chairs the annual conference on production machine learning (OpML). Nisha holds 68 patents in distributed systems and software, is a frequent speaker at industry and academic events, and is a contributing writer to Forbes Online/Cognitive World and other publications.
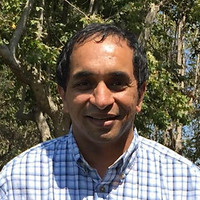
Dr.Amit Gupta
Amit Gupta is the Head of Products at AIClub. Amit has 20+ years of expertise in large-scale distributed systems design and development, and he was previously co-founder and CTO at Andale and Jasper. He holds a Ph.D. from the University of California at Berkeley, and has 50+ patents covering various aspects of Computer Networking, Distributed Systems, and Security.
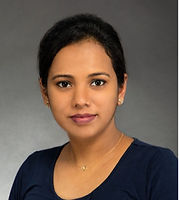
Dr.Sindhu Ghanta
Sindhu Ghanta received her M.S. degree from Texas Tech University in 2010 and her Ph.D. degree in electrical and computer engineering from Northeastern University, Boston, USA. She was a Post-Doctoral Fellow with BIDMC and the Department of Pathology, Harvard Medical School, where she was involved in detection and classification of features from histopathological images. She worked as a research scientist with Parallel Machines on monitoring the health of ML algorithms in production and has many publications on ML innovations. She currently works as the Head of Machine Learning at AIClub.

Learn about your mentors
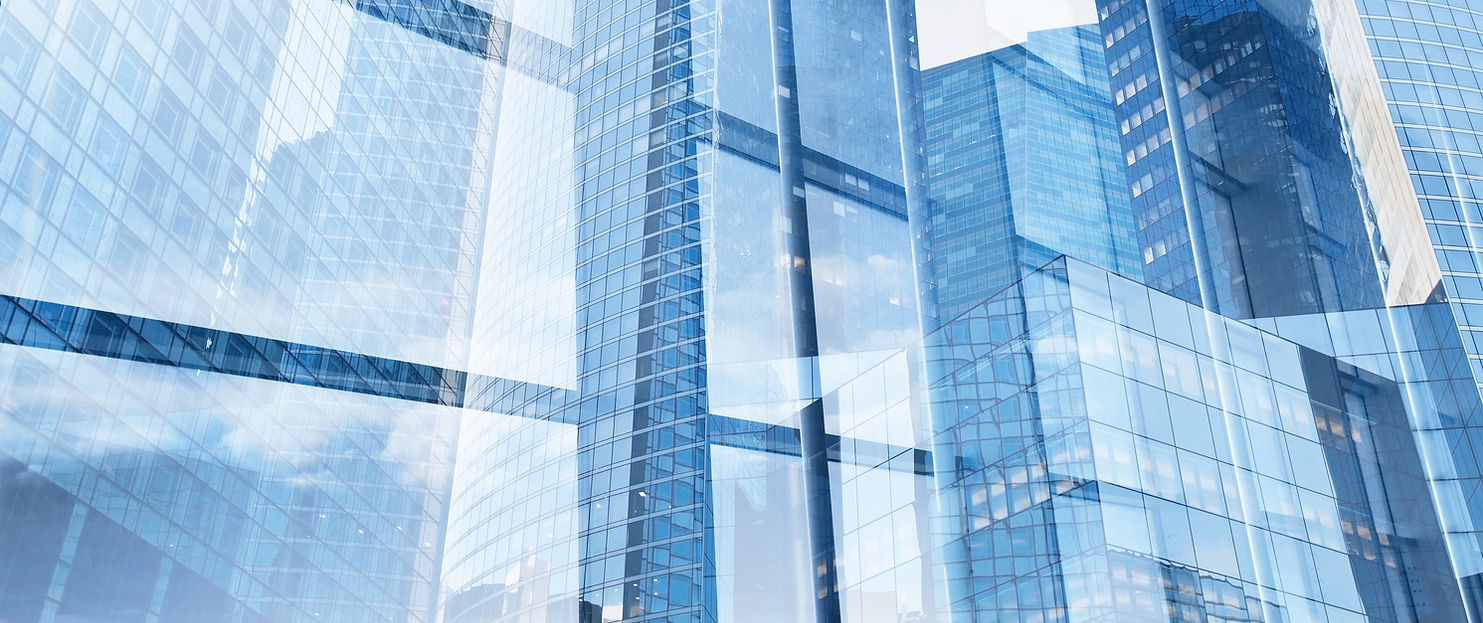
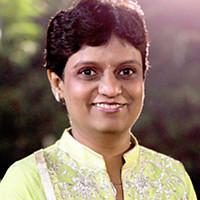
Dr.Smriti H. Bhandari
Smriti H. Bhandari is an accomplished researcher and educator with over 28 years of experience in Computer Vision, Machine Learning, and Database Systems. She holds a PhD in Computer Science and Engineering and has held leadership positions at prominent engineering colleges. Smriti has developed and delivered numerous courses and workshops in data science and deep learning, and served as the principal investigator on government-funded projects with applications in medicine and industry. She has received prestigious fellowships, including the Women Scientist and Young Scientist Fellowships, and has published extensively in her field. Smriti also brings industry experience as a freelance researcher and developer.

Dr.Vani Kandasamy
Vani Kandasamy has a PhD in Information and Communication Engineering from Anna University, Chennai. Her research is focused on applying machine learning techniques to analyze large-scale datasets, resulting in several publications in reputable journals and conferences. She has 10 years of teaching experience as an Assistant Professor at PSG College of Technology. During her tenure as an Assistant Professor, she developed and taught courses on topics such as Computer Vision, Natural Language Processing, Social Network Analysis and Data Privacy. Her ability to convey complex concepts in a clear and engaging manner, fostering a collaborative learning environment, has inspired students to excel in their studies.
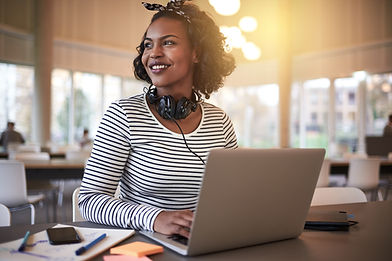
I cannot say enough about the AI Club and Ms. Nisha as a mentor. As parents we have been looking for what will spark our middle school son’s passion and interest. Within 6 months of being part of the AI Club, our son’s spark for AI and science blossomed. He went on to win first place and a special award for Symposium and now qualified for national and other selective science programs.
Bay Area Parents of 7th Grade Son
AIClub 1:1 FAQ
-
How does data help AI?Previously we talked a little bit about how AI's need a lot of data to learn patterns from. In this video we'll talk about how this data looks. Data can be in many forms, it can be numbers, images, videos, audio, etc. We will focus on data that can be stored in tables. You might have seen tables and softwares like Excel or Google Sheets. It essentially consists of some rows and some columns. What you see on this slide is that rows are numbered 1,2, 3 and so on, while the columns are labeled A, B, C etc. This is the basic structure of tables as you'll see in Google Sheets. It consists of several rows and columns. Let's take an example dataset. In this dataset, note that the columns are, number of countries visited, number of years in school, height and, who am I. You see how the two words feature and label appear right about in yellow color. These are really important 2 words. You'll be using them quite a lot when you build an AI. First thing to note is that there is a single column called label and rest everything is features. Label refers to what you would like your AI to predict and features represent information that you will provide your AI for it to make predictions. For example, in this data set, the features are `number of countries visited`, `number of years` in school and `height` and the label is `Who am I`. This means that you will give your AI information about how many years someone went to school, how many countries someone visited and their height. The AI will take this information and it is supposed to predict if this person is an adult or a child. Another word that you come across is sample. Every row here is called a sample. On this slide, for example, there are three samples,, which are provided to the AI to learn from. A really good question here is, what is a good number of samples? How many samples do you need to provide your AI, before it can learn to do predictions?. In the real world, there are sometimes millions of samples. It really depends on the kind of prediction that you're trying to make. The number of features which is the number of columns can also be like hundreds, or 1000s in the real world. For a simple example like this, probably a few 100 samples are enough for the AI to learn the pattern. But what happens if you don't provide enough samples?, what if I provide only these three samples?. The AI will have a really hard time to recognize patterns. So it may not do that great a job, if you don't provide enough samples. The process of building an AI can be divided into 2 stages. The first stage is called training. Training is the process by which AI learns patterns from data. For example, if you give it three features and one label column, it will try to recognize patterns in your features that will help it to predict the label. The output or result of training is something called a model. You can think about the model as the brain. This brain has learnt a specific task to recognize certain patterns in data and is now capable of making some predictions. Here is another example of providing data to an AI. This is a table which contains only two columns. The label column is the feeling, and the feature is the sentence. The AI will get this information as an input, and it is supposed to predict if someone is happy or sad. You can provide a bunch of these examples as data to your algorithm, and the algorithm will recognize patterns in these sentences and create a model. And once a model is created, it is capable of making predictions on new data that comes in. Now you can use this model in a second stage called the prediction stage where you can provide it with any English sentence or even a paragraph, and the AI is going to predict if that sentence is happy or sad. One thing to note is that an AI can do a very specific job, and how well it does that job depends on the quality of data that you provide. If you train an AI to detect if someone is happy or sad, then that's the only thing that AI can do. What will happen if you provided data about how many years someone went to school to an AI that has learnt to predict mood?. The AI has no idea about that kind of data, it will be super confused and do nothing. So AI's are very specific problem solvers, they learn to solve a specific problem. We have introduced a lot of new terms in this video. To reiterate, the new words that you have heard are training, which is the stage where you help your AI to learn patterns, prediction stage, which is also popularly called inference which is where your AI answers new questions. Lets talk about one last new term called AI Service. Going back to the example that we saw, where you can enter a sentence and it's going to predict happy or sad, a corresponding AI service would work something like this. You type in a sentence in english, lets say in a box and the AI service will take this as an input and predict if you're happy or sad. You actually interact with a lot of such AI services in your daily life, like Siri, google home, google translate etc. these are all AI services, where you have a model that has already trained on a lot of examples and is available for you to use. In this course, we are going to be creating such AI services, and depending on what kind of data you train your AI model with, the service is going to do a very specific job for you. So whenever we say AI service, think about it as the inference stage or the prediction stage, where you expect the service to accept new data and provide a prediction.